
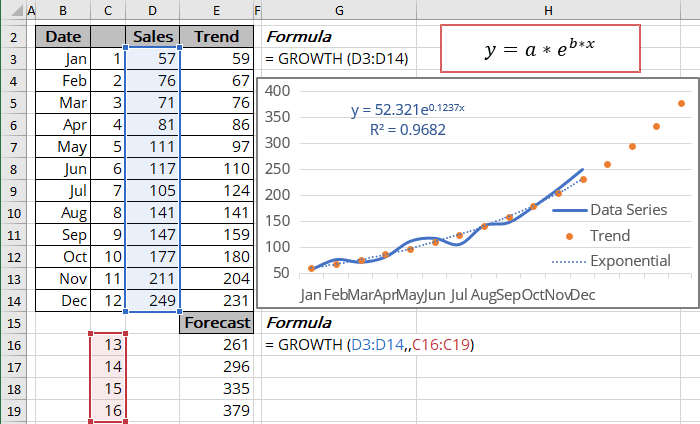
A popular neural net element computes a linear combination of its input signals, and applies a bounded logistic function as the activation function to the result this model can be seen as a "smoothed" variant of the classical threshold neuron.Ī common choice for the activation or "squashing" functions, used to clip for large magnitudes to keep the response of the neural network bounded is Logistic functions are often used in neural networks to introduce nonlinearity in the model or to clamp signals to within a specified interval. In particular, the Rasch model forms a basis for maximum likelihood estimation of the locations of objects or persons on a continuum, based on collections of categorical data, for example the abilities of persons on a continuum based on responses that have been categorized as correct and incorrect. A generalisation of the logistic function to multiple inputs is the softmax activation function, used in multinomial logistic regression.Īnother application of the logistic function is in the Rasch model, used in item response theory. Logistic regression and other log-linear models are also commonly used in machine learning. Where x x is the explanatory variable, a a and b b are model parameters to be fitted, and f f is the standard logistic function.

The standard logistic function, where L = 1, k = 1, x 0 = 0 A generalization of the logistic function is the hyperbolastic function of type I. The logistic function finds applications in a range of fields, including biology (especially ecology), biomathematics, chemistry, demography, economics, geoscience, mathematical psychology, probability, sociology, political science, linguistics, statistics, and artificial neural networks. įor values of x x in the domain of real numbers from − ∞ -\infty to + ∞ +\infty, the S-curve shown on the right is obtained, with the graph of f f approaching L L as x x approaches + ∞ +\infty and approaching zero as x x approaches − ∞ -\infty.


K k, the logistic growth rate or steepness of the curve.
